The Intersection of Big Data and AI: Unlocking New Frontiers
Businesses have long relied on data for decision-making. The volume and complexity of this data are growing exponentially, making it too difficult for traditional software and systems to process and analyze effectively.
At the same time, technological advancements have paved the way for the rise of artificial intelligence (AI), which has become a powerful tool for managing and analyzing big data. Big data and AI share a symbiotic relationship—AI tools depend on large data sets to learn and make predictions, while big data requires AI to make sense of its complexity. By understanding how these two forces work together, businesses can harness the power of AI to uncover real-time insights, optimize decision-making, and drive better results.
This blog is all about exploring the powerful collaboration between big data and AI, examining their individual roles, how they complement each other, and the challenges businesses face when implementing AI to harness the full potential of big data.
Big Data and AI: An Overview
Big data refers to bigger, more complicated data sets that are difficult for database tools to capture, manage, and analyze. It contains data from a variety of sources and activities. Big data, once accessed and understood, is incredibly beneficial to businesses because it allows you to make data-driven decisions that boost efficiency and profitability. However, interpreting and pulling insights from massive data brings unique obstacles. Big data is expanding in volume and velocity, and it contains both structured and unstructured data, making it difficult for existing data processing methods to handle.
This is where big data processing with AI technology comes in, enabling analytics tools to handle the expanding volume of big data. Artificial intelligence (AI) refers to computer science technologies that enable machines to accomplish tasks and replicate human capabilities. AI-based algorithms, natural language processing (NLP), and machine learning (ML) techniques are modeled after human decision-making processes, allowing computers to learn from data and draw acceptable conclusions and predictions. The use of AI in business operations has increased considerably, with many businesses reporting the use of AI in at least one business function.
Exploring the Relationship Between AI and Big Data
Artificial intelligence and big data are closely intertwined, each depending on the other to function effectively. AI needs vast data to enhance its learning and decision-making capabilities, while big data depends on AI-driven tools for efficient, precise analysis. Let us look at how these two collaborate:
Data Collection
Big data consists of structured data (e.g., numbers, text) and unstructured data (e.g., images, audio, video, or large text-based documents). Structured data is easy to store and process, while unstructured data is more challenging to organize. Both types come from customer interactions, social media, websites, devices, and sensors. This data powers AI tools, enabling them to process and learn, while AI applications help collect and organize big data more efficiently.
Data Storage and Processing
AI models need large, high-quality datasets for effective training, as limited or poor-quality data can compromise accuracy. Big data storage solutions, such as cloud-based data warehouses and data lakes, enable the storage and processing of vast amounts of data required for AI applications. Cloud-based storage boosts data transfer speed and minimizes bottlenecks. Additionally, businesses use big data's security and governance features to manage what AI can access for training.
Data Cleaning and Transformation
Big data is sourced from various platforms and needs to be pre-processed before AI can use it. This involves cleaning and structuring data for AI models. Modern cloud-based ETL(extract, transform, and load) technology extracts raw data, transforms it into a usable format, and loads it into databases for AI applications. AI technologies like NLP and ML are increasingly integrated into the ETL process to improve efficiency.
AI Modeling
Once data is processed, machine learning models use algorithms to analyze big data and make predictions or recognize patterns, with minimal human intervention. However, human oversight is necessary to validate predictions and to guide reinforcement learning.
Training Models
AI and ML models are only effective with sufficient training, which is where big data comes in. Models learn by accessing historical data and applying a specific algorithm to detect patterns in data based on the target, or answer, that you want the model to find. Several algorithms are frequently tested in models during training to determine which yields the best results. Once trained, a model can spot patterns and make predictions on previously unknown data in real-time.
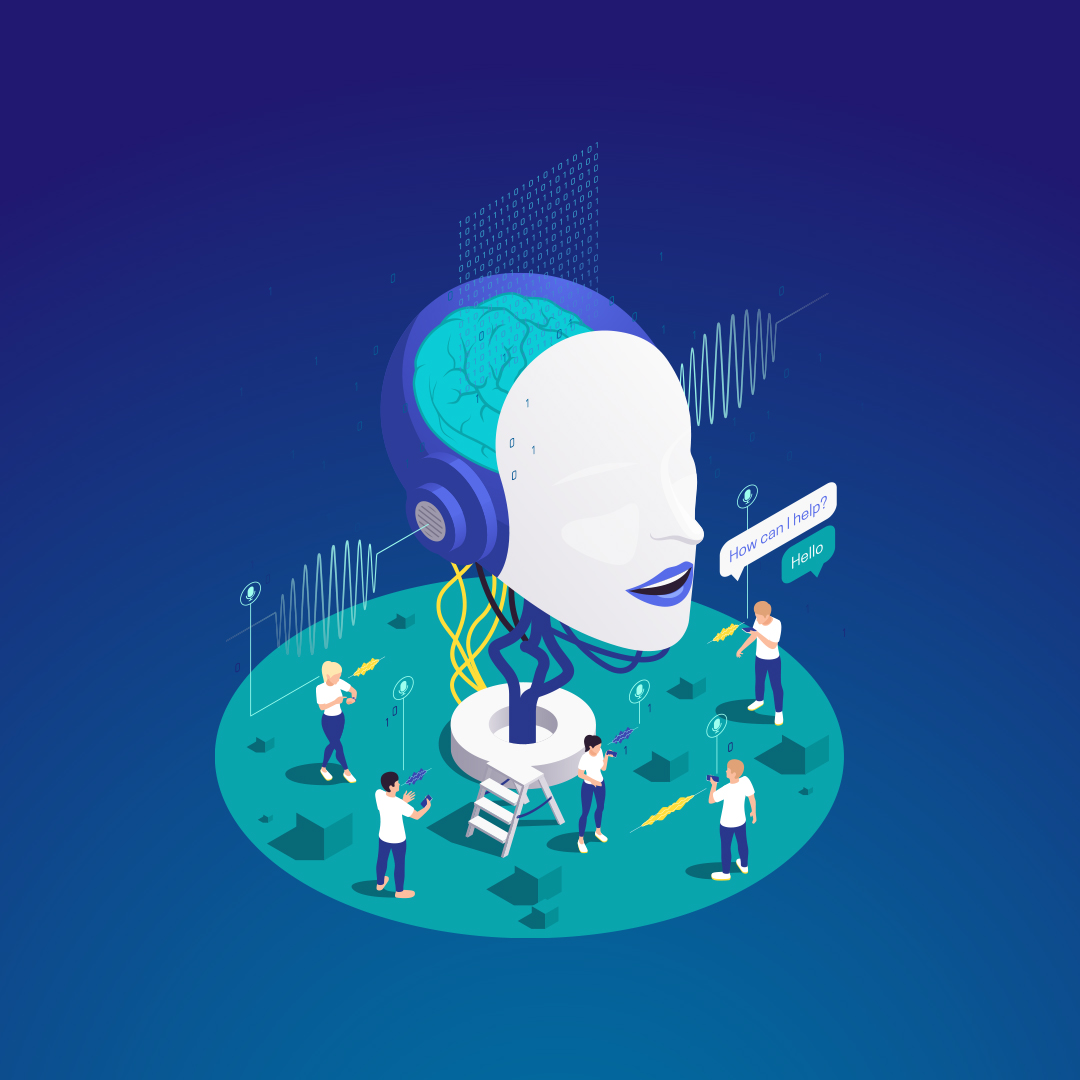
Generating Real-Time Insights
AI tools uncover trends, patterns, and anomalies in big data that humans might miss. Businesses can leverage these insights to improve operations, optimize services, adjust products, enhance security, and reduce risks. AI's data-driven predictions provide businesses with even more advantages, allowing them to design plans based on solid knowledge rather than gut feelings.
Innovating Each Other
AI and big data are closely intertwined, with AI relying on big data’s vast volumes and cloud storage to enhance decision-making. In turn, big data leverages AI techniques to maximize value. Innovations in one area boost efficiency and accuracy in the other.
AI-Driven Big Data Analytics: Top Use Cases
AI and big data help companies across industries make informed decisions. Here are a few key use cases:
Financial Services: In financial services, AI helps detect trends, risks, and fraud, while AI-powered chatbots assist with customer service. Machine learning models are used for credit risk assessment, and algorithm-based trading is also common.
Retail: In retail, AI predicts consumer trends to optimize inventory and drive product innovation. AI-powered data also helps create personalized recommendations for customers.
Marketing: In marketing, AI analyzes customer behavior and sentiment to segment audiences and deliver personalized campaigns based on preferences, engagement, interests, or purchase history.
Healthcare: In healthcare, AI analyzes patient charts, imaging, and other data to assist with diagnoses, create personalized treatment plans, and predict patient outcomes.
Manufacturing: In manufacturing, big data and AI improve quality control, reduce equipment downtime by predicting maintenance needs, and forecast trends affecting the supply chain.
IT: IT professionals use AI and big data to monitor tech infrastructure, predict maintenance requirements, and detect cybersecurity risks, helping to prevent attacks and minimize downtime.
Implementing AI for Big Data: Common Challenges
While the potential of big data and AI is immense, businesses exploring this dynamic field must be mindful of the challenges they may face. Here are some key hurdles to consider:
Data quality and quantity
Big data-powered AI solutions require high-quality, structured data in large quantities to function effectively. Poor data quality—such as inconsistencies, gaps, or bias—can skew results and lead to underperforming AI models.
Data privacy and security
Balancing data advantages with privacy and security is essential. Businesses must follow data privacy regulations and implement strong security measures to mitigate risks in data collection, storage, and analysis.
Finding skilled professionals
Securing professionals skilled in artificial intelligence, machine learning and big data processing is a significant challenge for businesses implementing AI solutions. Companies often face difficulties in attracting and retaining skilled professionals to manage these complex technologies effectively.
Building In-House Expertise
Training employees and fostering a data-driven culture is key to maximizing AI success. This may involve implementing training programs and reorganizing teams to ensure employees have the necessary skills to leverage AI effectively.
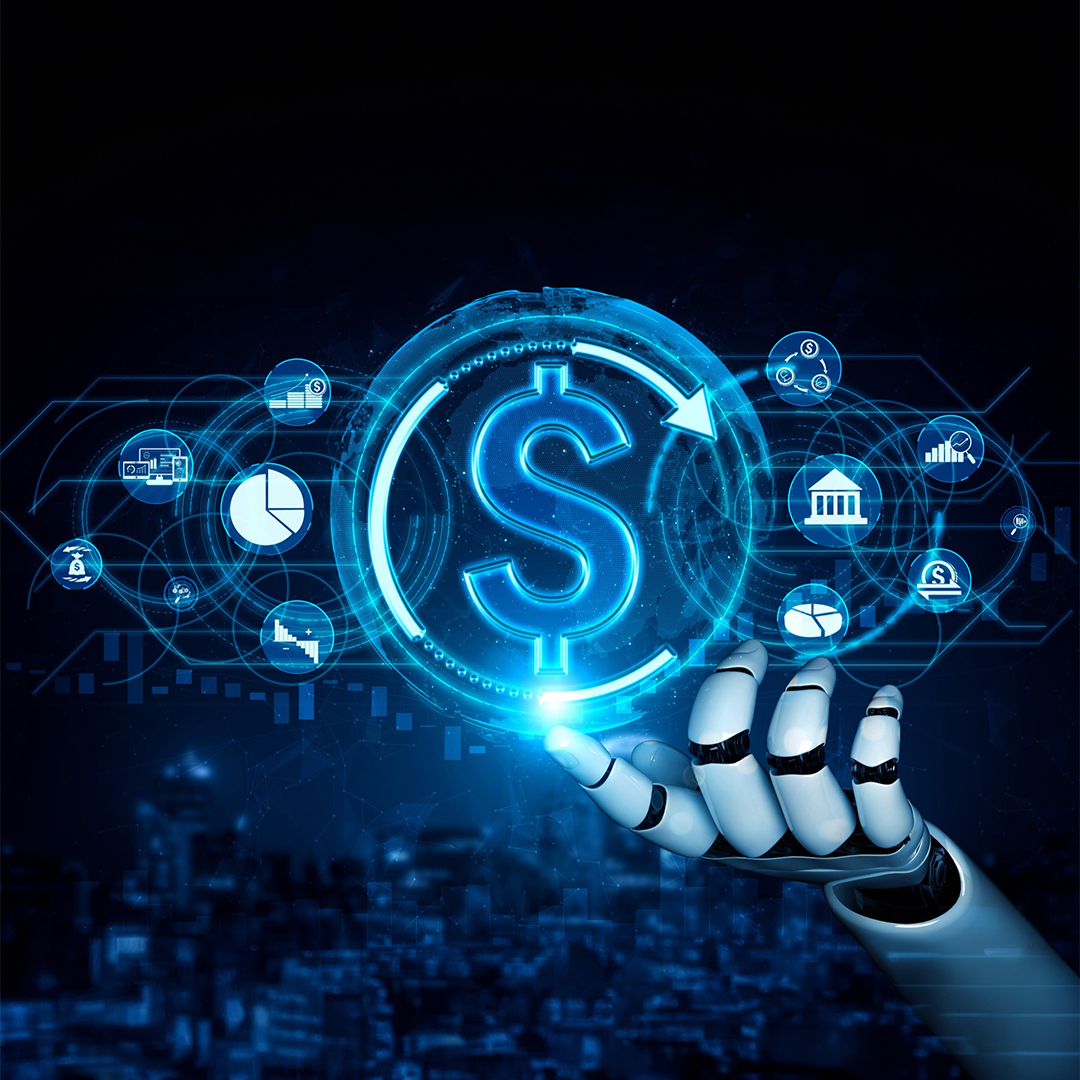
Investment in Technology and Resources
Developing and managing AI infrastructure can be difficult and costly. This includes purchasing hardware and software, as well as paying for cloud-based services.
Integration with Current Systems
Integrating AI technologies with existing systems can be a difficult undertaking that necessitates extensive technical skills. Data exchange agreements and compatibility are the most prevalent issues that firms encounter in this situation.
Unrealistic Expectations
Businesses may overestimate AI's capabilities and expect immediate outcomes. Setting reasonable expectations and acknowledging that AI is a strong tool but not a cure-all is critical.
Closing Thoughts
As AI advances and big data continues to grow, their synergy unlocks new possibilities for businesses to thrive in a data-driven world. Embracing this synergy can lead to a future in which enterprises not just survive, but thrive in a data-driven environment. The challenge is not whether to adopt AI for big data, but how quickly and effectively to embark on this transformative journey. Reach out now to get started!